Do You Even Decluster, Bro?
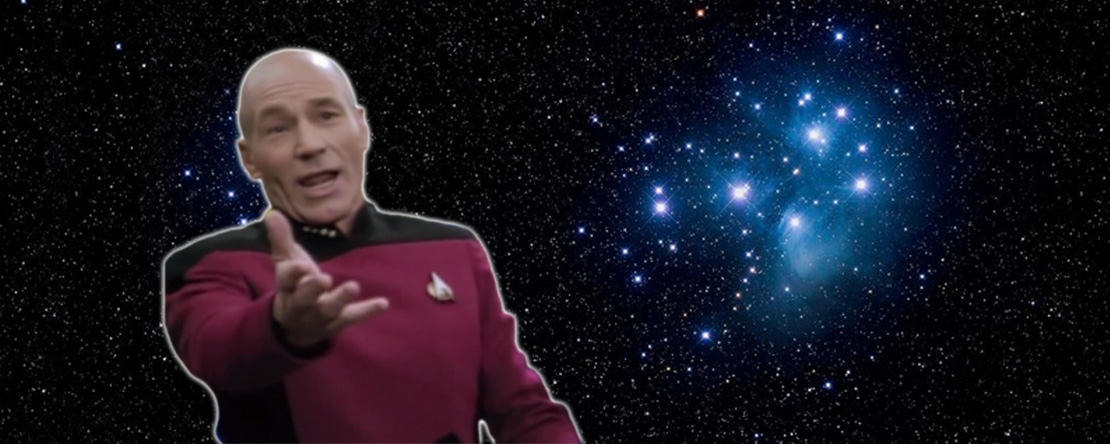
TL;DR
It is typical to collect data in a manner that preferentially samples high-valued areas over low-value areas. This preferential sampling is an acceptable practice; however, it produces closely spaced measurements that are statistically redundant, which results in areas that are under-represented by sparse data compared to the areas containing closer-spaced data when calculating statistics such as the global mean. This gives you statistics that are biased towards your closely spaced measurements. Therefore, it is desirable to have spatially representative (declustered) statistics for global resource assessment and to check estimated block models.
Based on my anecdotal evidence from reviewing many public/internal resource reports and talking to other resource geologists/engineers, declustering is often ignored or seen as a value-added step that you can do if you get around to it. However, it is critical to know the spatially representative mean when validating your estimated models, as your resource block model’s mean should approximate it. Moreover, considering declustering weights changes the shape of the distribution of the composites, so when you perform volume-variance checks on your model to see if you’re getting the right amount of smoothing or plot grade tonnage curves, declustering is an important step. Suppose you don’t have a declustered mean or declustering weights for these checks. In that case, you’re effectively using unrepresentative statistics to check a resource model that is supposed to represent the deposit. That sounds back-asswards… doesn’t it!?
Background
I feel like I’m going to reinvent the wheel if I blab on describing what declustering is and how you calculate declustering weights in this post. Instead, I want to dig into why we should care enough to go through the declustering workflow for our resource estimates.
If you need some background information, Ryan’s post introducing common data transformations gives an excellent introduction to what causes the need to decluster and provides a brief description of what declustering weights are. As for calculating the weights, naturally, there is a laundry list of methods you can use to calculate declustering weights… 🙄 Why can’t we have a silver bullet method to complete a task for once?! Regardless, the cell declustering method has proven itself as a reliable go-to method, as Clayton discusses in great detail and how you perform it.
Declustering is an essential step for uncertainty studies (MGK or simulations); however, to keep this post focused, I’m going to frame the discussion around resource studies that use an estimation method such as inverse distance or ordinary kriging. Also, I’m assuming data clustering occurs in high-grade areas and not in low-grade areas, flip the two in the discussion below if clustering in low-grade areas is the case you’d like to consider.
Spatially Representative Statistics
Most drill programs treat high-grade areas of the deposit like a pin-cushion by drilling the piss out of them, then avoid the areas with borderline economic grades (low-grade not waste) like the plague. The low grade-areas get drilled juuuust enough to make sure they get some inferred blocks in those areas. Then we can poke fun at the geologist that planned those dusty holes. It’s a win-win, really! Joking aside, this is an entirely reasonable approach compared to ensuring the deposit is evenly distributed with drillholes. The cost-benefit analysis of the uniformly distributed drilling will never stack up against the clustered drilling.
As a result of the clustered drilling, I think it is safe to say that most estimation domains will extend through those less drilled low-grade areas. For example, maybe you create a lower grade halo around a higher-grade core or link two high-grade shoots together with some lower-grade material. Either way, you’re grouping dense drilling with sparse drilling, which is the issue here. The estimation domain volumes are not evenly distributed with data, making any statistics you calculate with the raw composites biased towards the clustered data areas. Statistics calculated in this way aren’t “spatially representative,” which sounds like a mouthful, but it’s an important concept to wrap your head around.
I like to think of the ratio of data to the volume of rock within both the high-grade and low-grade areas to determine if I have clustered data, which may help some of you. For example, let’s assume you have an estimation domain that extends through the low-grade areas. If you were to visually assess the percentage of the estimation domain’s volume that you would classify as low-grade and high-grade (e.g., 50% LG, 50% HG), then guesstimate what percentage of your composites fall within each of those (e.g., 30% LG, 70% HG). Suppose the proportion of low-grade composites matched the low-grade volume, as with the high-grade ratio. In that case, you’re dealing with a situation where the as-is raw composites are approaching something you can maybe call “spatially representative.” This scenario isn’t the reality in most cases. The percentage of high-grade composites is most likely going to be higher than the percentage of the domain’s volume within the high-grade areas, which means the raw composites are not “spatially representative.” Therefore, you’re nearly always going to have to consider declustering.
In short, global statistics that are calculated using the raw composites are most likely not representative, as the composites within your domains are not evenly distributed within it. The volume of all the rock within the same domain must be treated with equal weight. We can’t cherry-pick our information to favour the high-grade areas within them. If this isn’t sitting well, you need to revisit how your estimation domains are constructed.
Why Is Declustering Not Widely Considered?
In the context of estimating a block model, calculating declustering weights isn’t a required preprocessing step, unlike simulation, which requires you to perform the normal score transformation on your declustered data. Not being required to perform declustering for estimation is likely a strong contributing factor to why it doesn’t receive all that much attention in resource studies. Well… that and the fact that the declustered mean is going to nearly always be smaller than the mean of the raw composites, making it a tough pill to swallow, and an even harder thing to explain to others when all they see you doing is making the mean of the composites smaller. It’s an ugly reality that we all need to deal with, but making sure we understand what the spatially representative statistics are will result in work that we know approximates the truth as best as we can using the currently available information.
Those of you out there that follow the CIM Estimation of Mineral Resources & Mineral Reserves Best Practice Guidelines, declustering is only mentioned twice, which is unfortunate, in my opinion. The document correctly suggests using declustering techniques to “get unbiased prediction of the global mean” so that you can “estimate unbiased grade tonnage curves.” While accurate, the brief recommendations make it hard to understand how vital declustering is for validation. If the process was expanded on and more strongly recommended, it might get some traction within the resource reporting world.
Dangers of Not Considering Declustering
Without a declustered mean, you have no way of validating the average grade of your block model. We know that the raw composite mean is biased, so if you’ve ever encountered a situation where your block model’s mean is much lower/higher then your raw composites, it could be wrong, it could be very wrong, or it could be right. However, you don’t know because you don’t know what the unbiased mean is that you should be approximating. That being said, declustering isn’t perfect, various methods will give different results, but the goal is to approximate the true unbiased mean of the deposit as best as we can.
When I see the mean of a global resource (measured, indicated, and inferred) without a cutoff applied that is very close to the raw composite mean, many red flags go off. I immediately know that some funny business has likely happened. Then the quality of the resource, at least in my mind, rapidly decreases unless I can find an explanation on why this occurs. Fortunately, estimation completed with reasonable search ellipsoids, variograms, capping, domains will prevent gross overestimation. I think it’s a realistic expectation of practitioners to document this simple validation. Without it, bad actors can fluff up a resource statement by slapping a block model with an unvalidated inflated mean into visually pleasing, albeit volume inflating bubble-wrapped grade shells that are posing as estimation domains. In other words, they put a nice thick layer of lipstick on that tiny pig that is far more complicated than once believed. Ring any bells?
For those of you out there that do volume-variance checks, you can use declustering weights when calculating the expected grade distribution given your mining block size so that it is spatially representative. If you’re not sure what volume-variance is, we’re sure to do a post on it very soon. It’s a critical validation step to ensure that the smoothing of your block model is right, given the size of your mining blocks. That way you know that you’re getting the right amount of blocks above the cutoff. This post here breaks the topic down very well, but it’s not for the faint of heart as it does get into the nitty-gritty. If formulas scare you off, there are a few sections in that post that summarise the topic well that you can cherry-pick.
And if that wasn’t enough! You should be using declustering weights when calculating grade-tonnage curves to make sure those are spatially representative.
Can I Even Use Declustering Weights, Bro?
The ability to use declustering in the way I’ve described will be very dependent on your software. I’d recommend contacting support for your software of choice and figure out where they allow you to consider declustering. Then you can compare your work with and without declustering. Make it so.
Additional Readings and Resources
- Rossi, M. E., & Deutsch, C. V. (2014). Mineral Resource Estimation. Edmonton AB: Springer Netherlands. https://doi.org/10.1007/978-1-4020-5717-5
- Michael Pyrcz videos on YouTube
- Deutsch, C. V. (2015). Cell Declustering Parameter Selection. In J. L. Deutsch (Ed.), Geostatistics Lessons. Retrieved from http://www.geostatisticslessons.com/lessons/celldeclustering
- Harding, B. E., & Deutsch, C. V. (2019). Change of Support and the Volume Variance Relation. In J. L. Deutsch (Ed.), Geostatistics Lessons. Retrieved from http://www.geostatisticslessons.com/lessons/changeofsupport